Have you witnessed a data science or analytics project built end-to-end by one person? This may have originally been a Proof of Concept (POC) in data science or, conversely, a solution that was actually operationalized.
If you have experienced this before, it's possible that there are two challenges you now face:
Who can help me if the individual that built this solution leaves? You are dealing with a one-to-one relationship between the solution and the person that can maintain it.
How was the solution built? You have limited knowledge on how the different datasets, preparations, and models are built and connected.
You have limited knowledge on what was done, how it was done, or how to support it moving forward which creates a significant amount of business risk to your data product. In this discussion, we’ll dive deeper into these challenges and their solutions.
Team Analytics
Collaboration on data science and analytics solutions is a must have for any organization that is serious about being data driven. As a consulting organization, we execute team analytics on every client project and you should too. Here’s why:
Faster ROI: Multiple people collaborating on solution construction leads to increased simplification and fewer misinterpretations of targeted business value. Example: “Maybe we don’t need to use deep learning if the sales team is asking for data-driven customer profiles.”
Fewer Mistakes: One misunderstanding of a data point can cause havoc on a machine learning model’s value. If more than one person is involved, you significantly decrease the chances you miss that minor detail that makes or breaks the model. Example: “Did ‘date’ mean requested date or delivery date? Oops.”
Decreased Risk: There is a significant amount of movement in the labor market with a forecasted end to the pandemic. This is especially true for roles in the data space. By involving more than one person in the solutions construction process, you lower your risk on the solution. Example: “Our data scientist that built and supported our pricing model took another job. Does anyone else know how to update it?”
Empowering Team Analytics
Now that we’ve identified the value of team analytics and collaboration, how do you empower that collaboration in practice?
We partner with Dataiku, whose end-to-end analytics features empower team analytics in many ways. I’d like to focus on three today.
End-to-End Transparency
A team needs to see a comprehensive picture of the analysis to understand what datasets and models are getting created and where preparation was done to create them.
Traceability
The below image is a screenshot that shows different data personas collaborating in a data science project. Each letter represents a different individual (A, V, S). You have two data scientists and a citizen data scientist working on a sales forecast model and a line efficiency model for a manufacturing production optimization effort.
This Dataiku feature shows how multiple personas participated in the solution and allows you to quickly trace who did what pieces of the solution.
Auditability
Change is inevitable. Dataiku’s out-of-the-box Git integration gives you the auditability and peace of mind you need to update solutions. Someone will eventually make a mistake. It’s a must-have feature to quickly roll back changes and save progress.
Team Analytics and Reduced Risk
By using a team on your analytics projects, rather than a single individual, you reduce risk to your efforts and produce better data solutions. The features in Dataiku empower teams to achieve the business value that collaboration provides.
Have your own example of how team analytics improved your analytics output? I’d love to hear it.
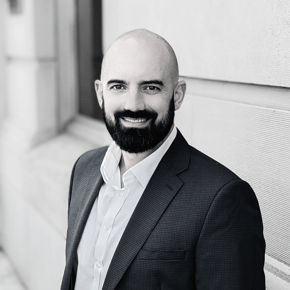